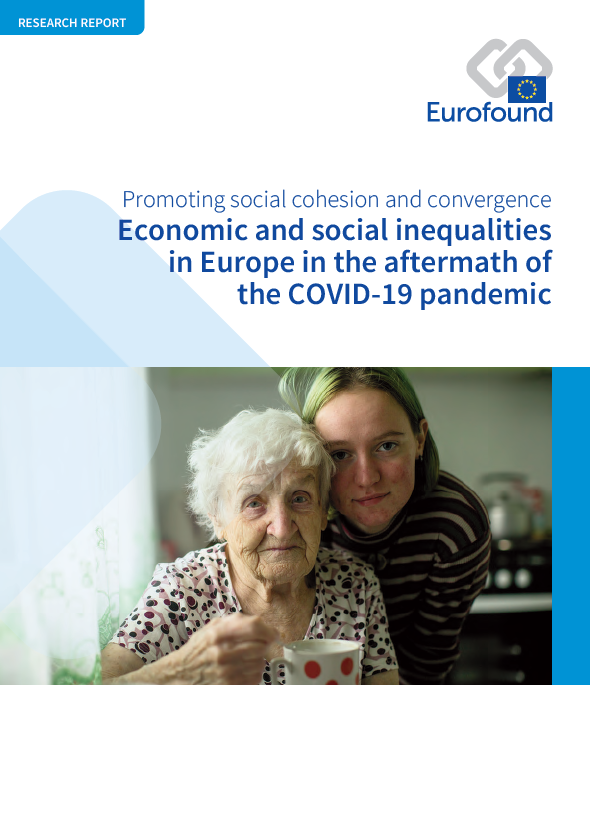
A pandemia de COVID-19 teve impactos variáveis nos grupos sociais, em função das desvantagens existentes, e é consensual que isso desencadeou um aumento das desigualdades em diferentes domínios da vida. Utilizando indicadores do Quadro de Monitorização das Desigualdades Multidimensionais na UE (MIMF) da UE, o presente relatório mostra como as desigualdades nos domínios dos rendimentos, da saúde, do emprego e da educação mudaram entre 2010 e 2020. Examina igualmente os principais fatores desta mudança durante a pandemia e explora as relações entre as políticas públicas em vários domínios e a desigualdade.
Key findings
No primeiro ano da crise da COVID-19, prosseguiu a queda das desigualdades de rendimentos, o que confirma um nivelamento das desigualdades na UE. No entanto, os candidatos a emprego e as pessoas com baixas ou médias habitações literárias foram os mais suscetíveis de sofrer uma diminuição dos rendimentos durante a pandemia, o que mostra que, embora a desigualdade de rendimentos em geral possa não ter aumentado durante a COVID-19, será fundamental que os decisores políticos acompanhem de perto esta situação na atual crise do custo de vida.
A saúde e a desigualdade de rendimentos estão estreitamente ligadas, sendo que as pessoas no quintil de rendimentos mais baixo são quase três vezes mais suscetíveis de sofrer de deficiência do que as pessoas nos 20 % mais elevados. Durante a pandemia, a desigualdade no acesso aos serviços de saúde em função dos rendimentos também aumentou: em 2020, o risco de necessidade médica não satisfeita nas pessoas no quintil de rendimento mais baixo era 5,4 vezes superior ao das pessoas nos 20 % mais elevados, o que destaca que as políticas centradas na redução das desigualdades de rendimento também podem reduzir as desigualdades no domínio da saúde.
Estudos revelam que o trabalho em casa durante a pandemia pode ter criado desigualdades entre grupos de baixos e elevados rendimentos, sendo que os trabalhadores temporários, os jovens e os trabalhadores precários se tornaram mais vulneráveis a crises. Para garantir que tal não se continue a verificar no mundo do trabalho cada vez mais flexível após a COVID-19, será crucial que os decisores políticos combatam o trabalho precário e aumentem a transparência e a previsibilidade das condições de trabalho.
Durante a pandemia, a existência de equipamento adequado para realizar a aprendizagem em linha foi mais importante do que os rendimentos, o que salienta a importância de combater o fosso digital e o acesso à tecnologia a longo prazo. Os pais e os alunos que vivem em zonas rurais, sem necessidade de se deslocar diariamente para o local de trabalho durante este período, também têm maior probabilidade de se mostrarem satisfeitos com a qualidade da escolaridade ou do ensino em linha do que os que vivem nas cidades.
A capacidade de trabalhar em casa criou desigualdades entre grupos com baixos e elevados rendimentos, acentuando a desigualdade de género no cuidado aos filhos e no trabalho doméstico. Em 2020, as mães solteiras foram mais suscetíveis de reduzir o seu horário de trabalho devido ao encerramento das escolas e estruturas de acolhimento de crianças — se as mulheres continuarem a trabalhar mais horas na prestação de cuidados não remunerados do que os homens, tal poderá aumentar a disparidade salarial entre homens e mulheres durante a recuperação.
The report contains the following lists of tables and figures.
List of tables
Table 1: Indicators selected for the income inequality analysis
Table 2: OLS regression model exploring the relationship between government spending and inequality in making ends meet according to education level
Table 3: Panel OLS regression exploring general drivers of income inequality (1995–2020), EU27
Table 4: OLS regression model exploring drivers of income inequality between rural and urban households
Table 5: OLS regression model exploring income inequality by individual characteristics
Table 6: Logistic regressions on income inequality by individual characteristics
Table 7: Indicators selected for the health inequality analysis
Table 8: OLS regression model exploring the relationship between government expenditure and inequality in chronic disease
Table 9: Multilevel logit regression model on worsening health between 2019 and 2020
Table 10: Multilevel logit regression models on worsening health and mental health between 2019 and 2020
Table 11: Indicators selected for the employment inequality analysis
Table 12: OLS regression model exploring the relationship between government expenditure and inequality in opportunity in having a white-collar job
Table 13: OLS regression model exploring the relationship between gender inequality in occupations, childcare and paid leave at country level
Table 14: OLS regression model exploring the relationship between gender inequality in being employed, childcare and paid leave at country level
Table 15: Random effects within–between model showing the relationship between gender inequality in employment, over time and between countries
Table 16: Multilevel linear regression model on the number of hours worked in 2019 and 2020
Table 17: Multilevel linear regression model on the change in the number of hours worked between 2018 and 2019 and between 2019 and 2020
Table 18: Indicators selected for inequality in education analysis
Table 19: OLS regression model exploring the relationship between government spending and inequality in PISA scores
Table 20: Determinants of respondents’ satisfaction with the quality of their children’s online schooling (multilevel ordered logit model)
List of figures
Figure 1: Dimensions of life of the EU MIMF
Figure 2: Intersectional approach to effects of COVID-19 on inequality
Figure 3: Macro-, meso- and micro-level factors in income inequality during the COVID-19 pandemic
Figure 4: Heatmap showing the results of income inequality indicators by country, 2018–2019, EU27 and the UK
Figure 5: Income quintile share ratio (S80/S20) for equivalised disposable income, EU27
Figure 6: Gini coefficient of equivalised disposable income, EU27, Bulgaria, Greece and Poland
Figure 7: Odds ratio of a household having problems making ends meet (with versus without a tertiary education, 2018) against spending on education (2015, % of GDP), EU27 and the UK
Figure 8: Odds ratio of a household having problems making ends meet (with versus without a tertiary education, 2018) against spending on social protection (2015, % of GDP), EU27 and the UK
Figure 9: Scatterplot of government spending on social protection (% of GDP at time t–1) relative to the Gini index of disposable income at time t (1995–2020), EU27
Figure 10: Odds ratio of households having problems making ends meet (rural versus urban, 2018) against public investments in agricultural R&D (2015, % of GDP), EU27 and the UK
Figure 11: Households that reported that their income decreased in 2020 compared with the previous year by country (%), selected Member States
Figure 12: Households containing people aged 50+ that received financial support from the government due to the pandemic by country (%), selected European countries
Figure 13: Recipients of pandemic-related government support by country, EU27 (%)
Figure 14: Macro-, meso- and micro-level factors in health inequality during the COVID-19 pandemic
Figure 15: Heatmap presenting the results of health inequality indicators, 2018–2019, EU27 and the UK
Figure 16: Map of odds ratios of people reporting unmet medical care needs (women versus men, adjusted), 2018
Figure 17: Heatmap of odds ratio of feeling depressed for different social groups, 2018–2019, EU27 and the UK
Figure 18: Risk ratios of having a severe long-standing limitation in usual activities (disability) due to a health problem for various social groups (2010–2020), EU27
Figure 19: Risk ratios of having an unmet medical need due to high cost, distance to travel or waiting lists for various social groups (2010–2020), EU27
Figure 20: Government spending on education in 2002 (% of GDP) relative to ex ante inequality of opportunity in having two or more chronic diseases in 2019 (aged 50+), EU27
Figure 21: Macro-, meso- and micro-level factors in inequality in working life outcomes during the COVID-19 pandemic
Figure 22: Heatmap showing results of working life inequality indicators, 2018–2019, EU27 and the UK
Figure 23: Risk ratios of gender inequality in various dimensions of working life (2002–2020), EU27
Figure 24: Risk ratios of unemployment rates among various social groups (2002–2020), EU27
Figure 25: Risk ratios of employment rates among various social groups (2002–2020), EU27
Figure 26: Odds ratio of women being in employment versus men (2019) against the share of children under three years of age in formal childcare (2019, %), EU27
Figure 27: Average number of weekly hours worked in 2020 by country and contract type, selected EU Member States
Figure 28: Proportion of women who held second or third jobs by household type, 2020 (%)
Figure 29: Macro-, meso- and micro-level factors in inequality in education and learning during the COVID-19 pandemic
Figure 30: Heatmap showing results of education inequality indicators, 2018–2019, EU27 and the UK
Figure 31: Difference in tertiary education attainment as a whole in 55- to 74-year-olds and those with parents with a lower than tertiary education (2021)
Figure 32: Trends regarding inequality in education between women and men (2002–2020), EU27
Figure 33: Risk and odds ratios of NEET rates between various social groups (2004–2020), EU27
Figure 34: Government spending on education (2013, % of GDP) against P90/P10 PISA scores in mathematics (2018), EU27 and the UK
Figure 35: Parents’ satisfaction with the quality of online schooling for their children, EU27 (%)
Figure 36: Parents’ satisfaction with the quality of their children’s online schooling depending on whether they worked from home or not during the pandemic, EU27 (%)
- Number of pages
-
102
- Reference nº
-
EF22002
- ISBN
-
978-92-897-2309-1
- Catalogue nº
-
TJ-07-23-019-EN-N
- DOI
-
10.2806/439913
- Permalink
Cite this publication
Eurofound (2023), Economic and social inequalities in Europe in the aftermath of the COVID-19 pandemic, Publications Office of the European Union, Luxembourg.