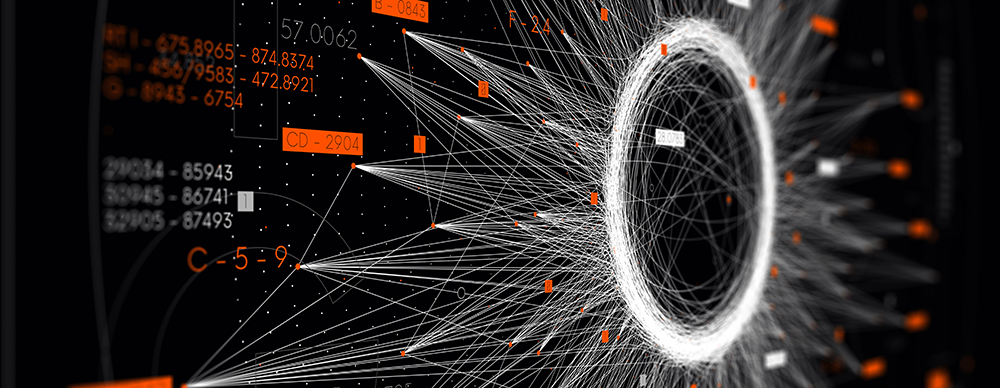
The EU High-Level Expert Group on Artificial Intelligence defines AI as follows:
Artificial intelligence (AI) systems are software (and possibly also hardware) systems designed by humans that, given a complex goal, act in the physical or digital dimension by perceiving their environment through data acquisition, interpreting the collected structured or unstructured data, reasoning on the knowledge, or processing the information, derived from this data and deciding the best action(s) to take to achieve the given goal. AI systems can either use symbolic rules or learn a numeric model, and they can also adapt their behaviour by analysing how the environment is affected by their previous actions.
As a scientific discipline, AI includes several approaches and techniques, such as machine learning (of which deep learning and reinforcement learning are specific examples), machine reasoning (which includes planning, scheduling, knowledge representation and reasoning, search, and optimization), and robotics (which includes control, perception, sensors and actuators, as well as the integration of all other techniques into cyber-physical systems).
A full list of references used to compile this research digest can be found at the end of the page.
Author: Eleonora Peruffo
Policy pointers
-
AI and the technologies supported by it can bring advantages to businesses and help companies to stay competitive at a global level. Fostering initiatives to promote the adoption of new technologies and related standards by small and medium-sized enterprises and bigger companies could contribute to keeping EU businesses competitive.
-
AI algorithms should be closely monitored to avoid bias and non-transparent decisions. The proposal for a regulation of the European Parliament and of the Council on machinery products (April 2021) could address harmful events deriving from the use of AI-empowered machinery, since it proposes that AI software ensuring the safety of machinery be independently vetted. Furthermore, as the AI workforce is highly gender-segregated, attracting more women into AI professions could be a powerful policy strategy to support ‘neutral AI’.
-
AI requires a digitally skilled and learning-oriented workforce; investing in digital and learning-to-learn competencies at all levels of the education system, including continuing professional development and lifelong learning, could ensure that the skills pool meets the demands of industry.
-
In terms of workers’ safety, training and awareness-raising on the functioning of AI-enabled machinery should be provided by employers.
-
Social dialogue can offer win–win solutions to challenges posed by AI, through the negotiation of transition measures or new forms of work organisation.
Digitalisation
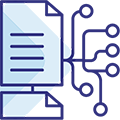
Introduction
Digitalisation increasingly permeates all aspects of life and the use of AI applications supports and accompanies this change. The COVID-19 pandemic has further accelerated digitalisation processes, prompting companies to shift to remote work or to automate certain processes to implement physical distancing. In healthcare, the positive aspects of AI manifested themselves in three main areas: for diagnostic imaging purposes; to explore possible combinations of drugs; and to extract information from patients’ data. In the world of work, AI can be used to improve and manage automation, digitisation processes and coordination through platforms.
The nature of AI as a general purpose technology – that is, a technology that can be applied to many sectors of the economy – makes it particularly relevant when studying the impact of technology on work and society. The concept of AI used in this research digest is that of ‘narrow AI’: machine learning and deep learning techniques. When talking about AI, it is useful to specify the field of application – for example, computer vision, speech recognition, deep learning, robotics and so on – and the specific implications it might have for workers and companies. Figure 1 shows the various areas of application of AI. Many studies and reports are not specific enough and group together different technologies (for example, AI, the internet of things, virtual reality), making it challenging to identify their impacts on employment and working conditions. This digest strives to underline differences between these technologies wherever possible.
Figure: Areas of application of AI

Source: Mills (2016)
At European level, the AI strategy links into and informs the wider EU digital strategy, which encompasses the General Data Protection Regulation, the Digital Skills Agenda to improve skills at all education levels, the Horizon Europe research programme, the Digital Markets Act and the Digital Services Act. The European Commission is leading several initiatives to develop AI research and foster the adoption of trustworthy AI – that is, AI where human supervision is built into the decision-making system – in accordance with the High-Level Expert Group on Artificial Intelligence’s Ethics guidelines for trustworthy AI, drawn up in 2019. In summary, AI should be:
- lawful: respecting all applicable laws and regulations
- ethical: respecting ethical principles and values
- robust: from a technical perspective, while considering its social environment
One of the most important principles is that AI processes and decisions should be subject to human supervision. In April 2021 these principles were included in the proposal for a regulation of the European Parliament and of the Council on machinery products. While recognising the usefulness of this technology, the proposal requires that AI-based software, and machines incorporating it, have conformity assessment certificates and that these assessments be vetted by an independent evaluator.
From a labour market perspective, data on the available workforce with AI skills (workers who have both statistical modelling and big data computational skills) are scarce but do indicate that the EU may employ half as many AI-skilled workers as the United States (US). The AI workforce is gender-skewed with only 16% of AI-skilled individuals being female. This raises some concerns regarding gender neutrality in the development and deployment of AI, including in the workplace.
Opportunities
- Improved use of human resources (HR) and operations through algorithmic matching
The digital age is characterised by the multiplication of data sources and the large volume of data available; the emergence of AI-based tools to gather, monitor, process and analyse these data presents an exceptional opportunity to improve products and services. Applications span sectors and occupations, but essentially the advantage brought by AI is the capacity to process a considerable volume of data at speed, making it possible to analyse data flows and gain useful insights for action. An example from the world of work is AI recruitment and hiring tools. These can facilitate CV screening and sorting, matching jobseekers with vacancies, scheduling job interviews and supporting online assessments, all with the ability to circumvent unconscious human bias, for example related to information on gender, age or ethnicity.
Therefore, AI is a strategic technology that can support the EU’s effort to transition to a digital society, benefiting both workers and companies in terms of health and safety, supporting competitiveness and improving processes.
AI and the technologies supported by it can bring advantages to businesses and help companies to stay competitive at a global level by improving their processes and reorganising the labour and capital balance. Fostering initiatives to promote the adoption of new technologies and related standards by small and medium-sized enterprises and bigger companies could contribute to keeping EU businesses competitive.
Risks
- Degradation of working conditions
- Loss of autonomy (algorithms could dictate pace of work)
Companies selling products that use AI processes could face liability challenges related to the way algorithms behave, especially if the algorithm in question is programmed to self-learn and optimise in a way that might lead to unforeseen circumstances, for example in self-driving cars. This has led the EU to publish guidelines on trustworthy AI. According to the White paper on artificial intelligence: A European approach to excellence and trust, the use of AI does not make products or services different from other non-AI-based ones, and consumer rights (data protection, privacy, non-discrimination) must be protected.
In the world of work, data are gathered not only about machines but also about workers; the ability to collect information about employees’ locations, activities and even bodies – for example, heart rate – and to analyse it in real time raises questions. Data insights could support the design of new Tayloristic forms of work organisation, including in platform work. As of mid-2021, debate was ongoing among social partners about workplace data ownership, the scope of their collection and the purposes for which the data can be used.
There is increasing concern about the impact of AI-based recruitment and algorithmic management when AI has been based or trained on biased data. This can result in reduced worker autonomy, risk of privacy violation, and bias and discrimination in task assignment and performance appraisal. Accordingly, there have been calls for caution and warnings about overreliance on AI in workplace-related decision-making.
From a societal point of view, ethics issues arise from algorithm-based decision-making. This is true of decision-making algorithms and also cases in which AI may trick humans into thinking they are dealing with other humans instead of a machine, for example AI assistants embedded in smartphones or other devices that can make phone calls on behalf of the user. During 2021, legislation on AI has been actively discussed at EU level. The debate focuses on how to classify algorithms and on liability. The EU white paper on AI proposes a risk-based approach, including the creation of notified bodies to carry out conformity assessments on AI-based software. Essentially, the proposal in the white paper points towards a sector-based approach to risk assessment, but many contributions to the debate emphasise that the approach should not be based on sector. Instead, a graded approach might be preferable, taking into consideration dependency and transparency, with the lowest level of risk requiring only analysis of suspicious cases and the highest level not permitted at all because of the high degree of damage that could be caused by non-transparent algorithms.
Concluding commentary
AI presents great opportunities but also great risks. Empirical evidence about job losses is still scarce. Previous catastrophic forecasts have not been borne out, although low-skilled workers have become more concentrated in highly automatable occupations, thus justifying calls for workforce upskilling to ensure that this group of workers is not left behind.
Balancing these two sides of the technology is the joint responsibility of government, employers and trade unions: AI is a tool – decisions on how to use it are up to humans. AI can potentially improve working conditions by reducing physical risks, but attention should be paid to emerging psychosocial risks.
Automation
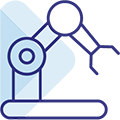
Automation is one of the ‘vectors of change’ identified as part of the broader notion of ‘digitalisation’ in Eurofound’s conceptual framework. It is the replacement of human input, in full or in part, by machine or software input. Advanced robotics, both for services and for manufacturing, is grouped with autonomous vehicles under the automation vector, since the ultimate aim of their application is to substitute machine for human input.
Examples of automation by AI are the replacement of workers on a production belt by an advanced robot, or the chatbots used in customer service, which replace human agents. Automation does not necessarily entail digitalisation – in the past, automation happened through mechanical means – but in the digital age automation is one of the fundamental drivers of digitalisation.
Introduction
Automation is at the centre of the debate about technological change. The topic has been a continuous thread spanning the centuries throughout industrial history, from Luddite protests to today’s concerns about applications of AI in finance, where algorithms are now in charge of high-frequency trading. The nature of automation has changed, however. While in previous times it was linked to mechanical routine tasks, since the beginning of the 21st century AI and other technologies have expanded the types of tasks that can be partially or entirely automated. Efforts to measure these potential changes are the subject of much debate at policy level. Automation, especially automation enabled by AI (advanced robots or software), can have an impact not only on the company undertaking it – transforming the balance between labour and capital inputs – but also on the workforce. Workers could be replaced by a machine and face the challenge of reallocating themselves in the economy or becoming unemployed. Suddenly, a matter specific to production process improvement can become a societal issue if it takes place at scale. The magnitude of the shift, however, has not yet been accurately measured.
Estimates of the extent of job replacement vary according to the methodology used. Early estimates pointed to half of US jobs being automatable; however, the authors of the study in question recognise that their analysis was limited because they considered jobs as monolithic units. In practice, jobs are bundles of tasks, and for an entire job to be considered automatable all the tasks involved in it would need to be automatable. This is not a common circumstance as most jobs entail non-routine cognitive tasks and tasks requiring social skills and emotional intelligence. Those tasks are one of the fields in which research on AI is taking place. Many challenges would need to be overcome for these tasks to be automated, relating, for example, to the availability of suitable training datasets and the capability of AI to recognise human emotions and to do so across cultures. Non-routine tasks are difficult to automate even when they involve manual work, for example the tasks performed by gardeners, plumbers and carers are not expected to be automated before 2030, and probably later than that.
Jobs that consist largely of routine tasks are more exposed to automation. These have been identified in postal services, agriculture, retail and clerical work.
Opportunities
- Improved business performance
- Improved working conditions
- Improved living conditions
For businesses in the manufacturing sector, AI can strengthen the operation of advanced robotics, on the one hand automating routine and standardised processes and on the other allowing flexibility of production through programmability. For workers, advanced robotics supported by AI can reduce their exposure to tasks that require physical effort, to hazardous machinery or situations, or to repetitive and standardised movements, thus reducing health and safety risks. The manufacturing sector is already highly automated, and robots are deployed mostly in three subsectors: automobiles; rubber and plastic; and metal products.
In services, AI could have an impact on many sectors, but this impact will differ depending on the characteristics of the service and the context in which AI is deployed. There is often a focus on the risks involved in task automation, but the opportunities presented are significant: the time freed up for workers could be dedicated to better interaction with customers, clients or patients. The list below showcases some examples.
- In healthcare, the use of AI to scan combinations of proteins has helped to create vaccines and to examine COVID-19 patients’ data to try to identify commonalities and differences. The pandemic has accelerated researchers’ interest in exploring the potential of this tool and finding useful applications. In other fields of application, such as radiology, cardiology and patient monitoring, AI means that workers have more time to analyse results, study complex cases and prepare treatments.
- The use of advanced robots to disinfect hospital wards is one of the possible ways in which technology could have a positive impact, although the investment required has been considered a barrier. With COVID-19, and the need to protect workers from becoming infected, such robots have been developed and are being adopted in hospital settings. The EU has provided EU hospitals with 200 robots that use UVC light to kill viruses. AI-enabled robots have other applications in healthcare, such as lifting and moving patients, thus reducing the risk of injury to workers.
- In customer services and sales, AI software increases response capacities by replacing customer calls with humans and handling chats using bots. Most queries can be handled by chatbots, allowing human agents to focus on non-standard queries and solve those problems.
- The use of AI to programme advanced robots can automate tasks previously requiring human action or supervision. For example, image recognition technology can help to recognise spoiled fruit and vegetables and eliminate them from the production belt.
- When carrying out maintenance in manufacturing, especially when the risk of physical harm is high, robots can be used to clean hard-to-reach areas or in proximity to hazardous substances.
- In public administration, processes involving the provision of services to citizens can be automated, for example to speed up processing of applications for benefits.
- In transport, AI is being used to control autonomous vehicles for civilian use, such as self-driving cars, platoon trucks and delivery drones. The optimistic forecasts of fast adoption of this technology in the early 2020s have, however, not yet been proved correct, because of technical and regulatory issues. Private or specially designated spaces such as port areas and campuses are the places where this technology is already being deployed. Rotterdam’s port retrained its truck drivers as autonomous vehicle supervisors, while in Berlin there has been a trial of a self-driving bus at the Charité University Hospital campus.
- In the hospitality industry, there is potential for AI-enhanced robots to perform the tasks of receptionists, waiters and cleaners. There have been some pilots, such as a hotel in Japan largely staffed by robots, but the level of AI needed to ensure a high standard of replies to customer queries is not yet available. Cleaning robots using AI-enabled computer vision find it challenging to navigate spaces such as hotel guests’ bedrooms, where objects may be left out of place.
- In rescue services, the use of AI-enhanced robots in hazardous situations reduces rescuers’ exposure to harm.
- In agriculture – a sector that is already highly automated but small in terms of number of workers – AI could offer opportunities for (further) automation of irrigation systems, harvesting and crop transport. This would imply less need for seasonal workers but probably result in an increase in demand for technicians able to maintain the machines. This sector is also likely to experience different impacts in different EU countries: advanced industrial farming conglomerates such as those in Germany and the Netherlands are likely to be early AI adopters, while individual farmers, found in particular in Bulgaria and Romania, are likely to lag behind.
- In various consultancy occupations – for example, health, legal and business consultancy – AI can assist the human expert in decision-making by analysing more data in a shorter period of time. This can lead to personal efficiency gains and upskilling as AI will cover the routine parts of the job while the human can focus on reviewing results, assessing them and communicating them.
Risks
- Algorithmic bias and reinforcement of (gender) stereotype
- Exploitation of monitoring/surveillance possibilities
The introduction of AI-related processes might constitute a reason for the reorganisation of companies. In some cases, the restructuring might entail a reallocation of resources, and in other cases the dismissal of workers whose tasks, and in some cases whole jobs, have been automated. Workers who do remain in employment after the introduction of AI need to be trained and made aware that AI machines are still prone to errors, and protocols have to be developed to avoid incidents.
A barrier to the introduction of more AI could be a lack of workers with the right digital skills. Not everyone needs to be able to programme algorithms – the trend is to make sophisticated calculations available to non-technical people through user-friendly interfaces – but a certain amount of digital savviness will be required of workers. As pointed out by the European Centre for the Development of Vocational Training (Cedefop), by forcing people to move their activities online as much as possible, the COVID-19 pandemic exposed the demand for, and lack of, digital skills. This increased demand is set to continue, since companies have changed business models and digital skills are becoming a requirement in all sectors and occupations.
For society, the automation of processes in various sectors – including the transport sector, with the possible availability of self-driving vehicles – might pose challenges. If a substantial number of workers displaced by the technology cannot find a new job because they lack the skills needed for jobs created by the introduction of AI, there could be repercussions such as a rise in unemployment and public discontent.
Furthermore, the automation of processes is not completely separate from digitisation; often, automation takes place after data have been collected and used to inform the process and train algorithms. However, the importance of data collection goes beyond the training stage, since many algorithms can be improved by continuous data collection. The privacy of passengers (for example, in buses or taxis) and passers-by should be protected by design, and it should be clear to customers what data are being collected and how they will be used.
The delegation of decisions to algorithms (for example, on unemployment benefit eligibility or ranking on a waiting list for social housing) has both positive and negative sides. The algorithmic automation of lengthy processes could result in faster provision of services, but it could also present risks if it is not implemented in a transparent and fair manner. Evidence from academic studies and real-life examples indicates that algorithms should be trained on datasets that are not biased and the use of non-‘black box’ methods preferred. When algorithms use black-box methods, for example neural networks, it is very difficult to audit all the steps that bring the algorithm to a particular decision or result. As of July 2021, data science was making progress on developing ways to unearth these hidden processes. Automated decisions, as indicated by the EU guidelines on trustworthy AI, should always be made with human supervision. This applies to public services application processes as well as HR decisions in the workplace.
Concluding commentary
The use of AI for automation brings clear opportunities for companies to increase their competitiveness and at the same time improve working conditions, especially in terms of health and safety. The risks associated with it are not negligible, however. A continuous exchange of information and views among stakeholders, along with appropriate regulation and its enforcement, could help to mitigate the impact of biased algorithms, to limit extreme employee surveillance and to make sure that ultimate decisions include a ‘human in the loop’.
The introduction of AI into the workplace and its consequences can be managed so that work organisation decisions do not excessively penalise workers. Social partners can negotiate upskilling programmes and other solutions that can save jobs and benefit companies.
AI requires a digitally skilled and learning-oriented workforce; investing in digital and learning-to-learn competencies at all levels of the education system, including continuing professional development and lifelong learning, could ensure that the skills pool meets the demands of industry. Solutions such as upskilling programmes and unemployment support could help in tackling unemployment challenges. Furthermore, women, people with a migrant background and people with disabilities could be attracted to train and work in AI by, for example, tackling gender stereotypes in education and the labour market. Nudging workers to acquire skills not easily replaceable by AI (for example, creativity, social skills, reasoning, decision-making in uncertain environments) would also be one way to help workers to adapt to work organisation changes. The shift to AI is also linked to cost-effectiveness compared with human labour; where human labour is more cost-effective, automation is not a predetermined outcome.
Automation is driving changes in people’s tasks, and sometimes in their entire jobs, which could cause job displacement unless social partners agree on new forms of work organisation or transition support measures. Even more importantly, decision-makers could influence which jobs are automated and which are not, especially in cases where human interaction is needed or could have a positive effect, for example in healthcare. The answer that the EU gives to these questions will contribute to determining the extent to which and how AI shapes society: yes, it can standardise processes and make them cheaper, but what about activities such as hospitality? The question of how operators in hospitality are going to differentiate themselves from competitors if everything is handled by machines remains open. And, perhaps more importantly, when AI reaches a point where interaction is rewarding for humans, can the education of young minds be left to robots and algorithms, or should human interaction be considered a fundamental part of learning and growing up? These issues are still to be decided on, and governments and social partners can play a part by continuing the debate and informing AI developments, as was the case in the development of principles on trustworthy AI.
The impact of AI varies across sectors and EU countries because its impact depends on the number of people employed in a sector, on the level of automation in a sector and on the automatability of tasks.
Digitisation
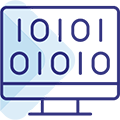
Digitisation is one of the ‘vectors of change’ forming part of ‘digitalisation’ in Eurofound’s conceptual framework. It refers to the process through which aspects of the physical world are rendered into data and virtual models, and vice versa. Three main technologies fall under this vector of change, namely 3D printing, augmented and virtual reality, and the internet of things.
AI supports the effective manipulation and interpretation of digitised data and information.
Introduction
Along with automation, digitisation is one of the main drivers of the digital age. The amount of data produced per day now exceeds 2.5 quintillion bytes (2.5 × 1018). This includes not only data produced by users online but also manufacturing data, telecommunications data and so on. In the world of work, the gathering, processing and analysis of these data can help companies to stay competitive in their domain. The implementation of digitised processes can transform working conditions, for better or for worse, by altering jobs and tasks. At a societal level, the availability of data means that digitisation can bring advantages to citizens’ lives. These can take the form of smart homes or smart cities, for example; however, it must not be forgotten that AI-based algorithms should respect people’s privacy.
Opportunities
- Faster processing of information
- Data insights: wider information basis for business and workplace decisions
- Potential for remote control of cyberfactories
- Improved preventive maintenance
- Use of algorithms for fraud detection
For companies, there are opportunities in the use of AI that can be looked at through a sectoral lens for specific impacts and at a process level for practices, such as HR, fraud detection and cybersecurity, that are not industry specific. This implies that companies that want to use AI need to train or hire employees with expertise in machine learning or deep learning. Perhaps one of the most cited examples of business transformation through AI is online retail, where the use of algorithms to identify customers’ behaviour and buying patterns and to suggest products to customers is already established.
In the manufacturing sector, the digital age transformation of production processes is often referred to as ‘Industry 4.0’ (or ‘advanced manufacturing’ in the US). Industry 4.0 is a concept that spans automation and digitisation and alludes to factories becoming cyber-physical spaces, to the point where a ‘virtual twin’ of the factory allows many processes to be controlled remotely. These types of applications are already very advanced in the aviation industry (for example, the Airbus A350 has 50,000 sensors on board, collecting 2.5 terabytes of data every day). The reduction in the cost of sensors offers opportunities to companies in other industries, too. The possibility of monitoring appliances beyond the production phase and beyond their point of sale is called the ‘servitisation’ of manufacturing. The use of sensors enables the manufacturer to monitor appliances’ performance, providing input into future design, and to offer prompt intervention in the event of failure.
A cyber-physical workspace has implications for work organisation and workers. First, if most of the work is automated and digitally monitored, there are fewer workers on the shop floor and more workers doing monitoring and maintenance, and there is a need for experts in engineering and mechatronics, data analysts and cybersecurity experts. Second, an advanced virtual twin factory can be monitored from afar, which means that the controller could be based far away from it, in another country or on another continent. Third, if most processes depend on machines or robots, the factory can run 24/7, with no downtime except for maintenance. The use of predictive algorithms means that maintenance itself is set to become more and more a scheduled event rather than an unexpected occurrence, since AI can identify signal patterns indicating that if action is not taken breakdown is likely, thus enabling early intervention.
In healthcare, during the COVID-19 pandemic, the possibility of checking many combinations of proteins against patient data to find the right drugs showed the importance of powerful AI algorithms. Monitoring patients from a distance is another way in which the digitisation of inputs using sensors can present opportunities to rethink some of the tasks performed by carers and at the same time gain accurate readings on patients’ health.
The potential of digital information can be used in training, for example virtual reality can reproduce high-risk and hazardous environments to help in training rescue workers or firefighters). An exercise can be repeated as many times as necessary, and analysis of the trainees’ movements using AI can help to improve both the training and the software. Augmented reality could help in upskilling workers, for example using smart glasses to superimpose instructions on an object that needs to be fixed.
Risks
- Exploitation of monitoring/surveillance possibilities
- Data theft/data breaches
- Weak cybersecurity
- Loss of autonomy (algorithms could dictate pace of work)
Remote control of cyberfactories could have consequences for employment and working conditions. First, if monitoring of production processes can be done remotely, this implies that jobs can be moved to the cheapest possible location, not at local or national level but at global level. Second, some applications of AI, such as augmented reality, could decrease workers’ autonomy, as some workers may receive instructions from machines (via interfaces), and the control of systems over some tasks may give rise to a feeling of alienation. In terms of working time, there could be a decrease in the quality of working life as a result of the 24/7 nature of cyberfactories and the need to be on call.
The collection and analysis of digitised information also have implications for the monitoring of workers, which in extreme cases becomes surveillance. Where work can be performed remotely through ICT, AI can potentially be used to conduct digital surveillance of workers. Surveillance could also occur on the shop floor, for example where sensors must be worn by workers to monitor positions or body functions, such as heart rate, for health and safety purposes. These data should be collected only for the intended purpose of protecting workers’ health and safety and not to gain further insights or be sold. AI devices could also enable data collection about workers gathering together, the frequency of their interactions, the number of breaks they take and the duration of rest periods; data could also be used to score workers’ performance and determine their pay. All of this could reduce workers’ privacy and harm their psychosocial well-being. Intense surveillance could lead to performance-driven remuneration, which would have an impact on working time and workers’ rights; for example, it could result in workers not taking sick leave or using holidays.
Concluding commentary
AI is a tool and, as such, what matters is how it is applied by companies. Work organisation can play a role in creating win–win outcomes for employers and workers, improving productivity, competitiveness and working conditions, and respecting workers’ privacy. Social dialogue can play an important part in the design and implementation of these technologies.
In the workplace, it should be ensured that data generated from the interaction of digitisation and AI technologies are collected only for the intended purpose of protecting workers’ health and safety, and not to gain further insights or be sold.
Platforms
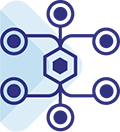
Platform work is a form of employment in which organisations or individuals use an online platform to access other organisations or individuals to solve problems or provide services in exchange for payment.
Introduction
Despite its small scale – around 1–2% of the EU workforce does it as a main job – platform work is classified as one of the nine emerging forms of employment. The central mechanism of platform work is the capability to centrally and algorithmically match supply of and demand for labour, and in some instances, also manage workers’ tasks. Communication with workers takes place through a digital device – a computer or, if the worker is performing a task that entails geospatial movement, a smartphone. Workers’ tasks can be rated by users of the service. In certain cases, the rating depends on speed, efficiency and other relevant aspects of the task, calculated by an algorithm. The ratings determine the ‘success’ of the worker in obtaining new assignments and/or the characteristics of the assignments, such as their scale, price or quality; a batch of bad ratings could lead to a worker losing access to work as a result of (algorithmically established) underperformance.
One of the issues raised by platform work is that the rules used to inform the algorithms – which are designed by the platform – are frequently not communicated to the workers. Such an algorithm is often referred to as being a ‘black box’ for the worker because of the lack of transparency of its characteristics/mechanisms. Even if the rules are known, there is very little space for the worker to negotiate in relation to a task – something that would be at least a possibility if a human supervisor were involved in the decision. An example might be the case of a worker who cannot sign in to the platform or complete a task because of an issue that has suddenly arisen. Another example would be an instance where a delivery rider has always had the same shift, and then suddenly the shift is no longer available, without any explanation from the platform.
Opportunities
Platform work presents advantages for clients because it provides real-time matching services supported by AI. For companies using it as a work organisation tool, it also allows the algorithmic automation of certain aspects of HR management, such as scheduling and assignment of tasks.
In theory, algorithmic matching can overcome human bias (discrimination) in selecting service providers as, if the algorithm is well designed, it does not base decisions on stereotypes related to, for example, the gender, age or ethnic background of the platform worker. This can be to the benefit of vulnerable groups on the labour market.
For professionals using platforms as a means – sometimes an additional means – of finding clients, algorithmic matching can reduce the time taken to identify and approach clients, and hence increase productivity.
Risks
- Degradation of working conditions
- Loss of autonomy (algorithms could dictate pace of work)
The non-transparency of algorithms makes it hard for the worker to adapt to the ‘ideal’ way of working as understood by the platform. Even when the algorithmic rules are known, there is little scope for negotiation. As of 2021, whether algorithms tend to be more neutral or even more prone to discrimination than a human task assigner is still to be proved.
Where a platform/algorithm is very intrusive in terms of determining work organisation and working conditions, research finds rather negative outcomes for workers (in terms of working time, work quality, income, autonomy, career development/learning opportunities and so on).
Concluding commentary
While algorithmic coordination presents undeniable advantages in terms of matching services and, for companies, efficient scheduling and task assignment, for workers the negative implications may outweigh the positive ones in those types of platform work where algorithms have a powerful role. AI can offer many solutions and writing algorithms that use rules agreed upon between the platform and its workers could be a way forward.
Related material
Related policy pointers | Related research digests |
---|---|
References
References
Eurofound sources
Eurofound (2016), What do Europeans do at work? A task-based analysis: European Jobs Monitor 2016 , Publications Office of the European Union, Luxembourg.
Eurofound (2018), Automation, digitisation and platforms: Implications for work and employment , Publications Office of the European Union, Luxembourg.
Eurofound (2019), Autonomous transport devices: Implications of game-changing technologies in the services sector in Europe , Eurofound working paper, Dublin.
Eurofound (2020), Employee monitoring and surveillance: The challenges of digitalisation , Publications Office of the European Union, Luxembourg.
Eurofound (2020), Game-changing technologies: Transforming production and employment in Europe , Publications Office of the European Union, Luxembourg.
European Union and Eurofound (2019), How computerisation is transforming jobs: Evidence from Eurofound’s European Working Conditions Survey , European Commission, Seville.
Other sources
Airbus (undated), Data revolution in aviation , web page, accessed 17 September 2021.
Arntz, M., Gregory, T. and Zierahn, U. (2016), The risk of automation for jobs in OECD countries: A comparative analysis , OECD Social, Employment and Migration Working Papers No. 189, OECD Publishing, Paris.
Autor, D. H. (2013), The ‘task approach’ to labour markets: An overview , Working Paper No. 18711, National Bureau of Economic Research, Cambridge, Massachusetts.
Brynjolfsson, E. and McAfee, A. (2011), The second machine age, W. W. Norton & Company, New York.
Cedefop (2021), Trends, transitions and transformation: The Covid-19 pandemic is challenging skill needs and reshaping jobs, while challenging our understanding and analysis of them , briefing note, Thessaloniki.
Deshpande, A., Picken, N., Kunertova, L., de Silva, A., Lanfredi, G. and Hofman, J. (2021), Improving working conditions using artificial intelligence , publication for the Special Committee on Artificial Intelligence in a Digital Age, Policy Department for Economic, Scientific and Quality of Life Policies, European Parliament, Luxembourg.
European Commission (2021), A European approach to artificial intelligence , web page, accessed 17 September 2021.
European Commission (2021), ‘ Coronavirus: Commission to provide 200 disinfection robots to European hospitals ’, press release, 8 March.
European Commission (2021), Proposal for a Regulation of the European Parliament and of the Council on machinery products , COM(2021)202 final, Brussels.
European Institute for Gender Equality (forthcoming), Artificial intelligence, platform work and gender equality, Publications Office of the European Union, Luxembourg.
Frey, C. F. and Osborne, M. A. (2017), ‘ The future of employment: How susceptible are jobs to computerisation ? ’ Technological Forecasting and Social Change, Vol. 114, pp. 254–280.
Georgieff, A. and Milanez, A. (2021), What happened to jobs at high risk of automation? OECD Social, Employment and Migration Working Papers No. 255, OECD Publishing, Paris.
Harmon, S., Sanford, T. and Turkbey, B. (2020), ‘ Artificial intelligence for the detection of COVID-19 pneumonia on chest CT using multinational datasets ’, Nature Communications, Vol. 11, No. 4080.
High-Level Expert Group on Artificial Intelligence (2019), Ethics guidelines for trustworthy AI , European Commission, Brussels.
IEEE Spectrum (2020), ‘ What AI can – and can’t – do in the race for a coronavirus vaccine ’, blog post, 29 September.
LinkedIn (2019), AI talent in the European labour market , web page, accessed 17 September 2021.
Mills, N. (2016), Artificial intelligence in law: The state of play 2016, Thomson Reuters Legal Executive Institute, Toronto.
Nedelkoska, L. and Quintini, G. (2018), Automation, skills use and training , OECD Social, Employment and Migration Working Papers No. 202, OECD Publishing, Paris.
Raconteur (2020), ‘ Inside the hotel run by robots ’, blog post, 19 April.
Scientific Foresight Unit, European Parliamentary Research Service (2021), Digital automation and the future of work , Brussels.
The Guardian (2015), ‘ Japan’s robot hotel: A dinosaur at reception, a machine for room service ’, news article, 16 July.
Image © max_776/Adobe Stock Photos